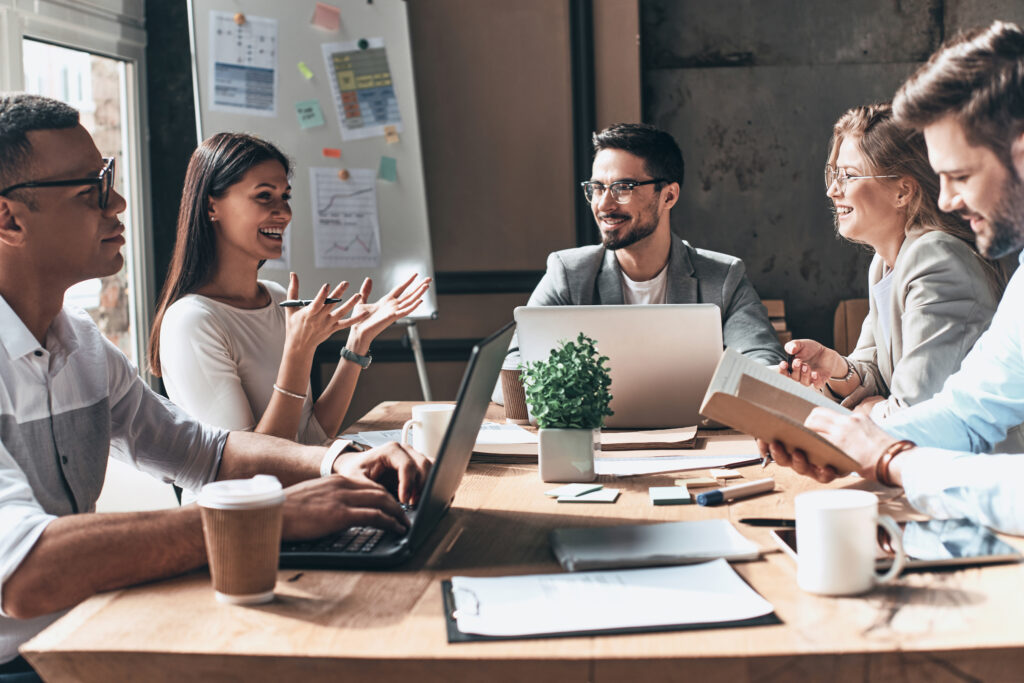
The relationship between sales and marketing has always been complex—sometimes collaborative, sometimes contentious, but always critical to business success. Today, as artificial intelligence transforms both functions, this relationship is evolving once again. For marketing teams, understanding how AI is reshaping sales enablement isn’t just helpful—it’s essential for maintaining relevance and driving revenue in the modern business landscape.
The Evolving Definition of Sales Enablement
Sales enablement has traditionally focused on equipping sales teams with the content, tools, and information needed to sell effectively. In the AI era, this definition has expanded dramatically:
Traditional sales enablement centered on:
- Static sales collateral and battle cards
- Periodic training sessions
- Basic CRM systems
- Standardized messaging
AI-powered sales enablement now encompasses:
- Dynamic, personalized content delivery
- Real-time coaching and guidance
- Predictive analytics and buyer insights
- Automated workflow optimization
- Continuous skill development
This evolution creates new imperatives for marketing teams supporting the sales function. Understanding these changes is the first step toward effective collaboration in the AI-enabled sales ecosystem.
How AI is Transforming the Sales Function
Before examining marketing’s role, it’s important to understand how AI is reshaping sales operations and capabilities:
1. Hyper-Personalization at Scale
AI enables sales teams to customize their approach for each prospect without sacrificing efficiency:
- Personalized outreach: AI analyzes prospect data to suggest tailored messaging
- Dynamic presentation customization: Automatically adjusting pitch decks based on buyer signals
- Individualized follow-up sequences: Creating custom nurture paths based on engagement patterns
Impact on sales behavior: Sales representatives increasingly expect personalization capabilities in their enablement tools, requiring marketing to move beyond one-size-fits-all assets.
2. Predictive Engagement Models
AI helps sales teams understand not just who to target but when and how:
- Optimal timing recommendations: Suggesting the best times to engage specific prospects
- Channel preference prediction: Identifying which communication methods will be most effective
- Next-best-action guidance: Recommending logical next steps based on buyer behavior
Impact on sales behavior: Sales teams now operate more strategically, requiring marketing to provide content appropriate for specific buying stages and engagement scenarios.
3. Conversational Intelligence
AI provides unprecedented visibility into sales conversations:
- Call and meeting analysis: Automatically identifying successful talk tracks and objection handling
- Buyer sentiment detection: Gauging prospect receptiveness and concerns in real time
- Competitive intelligence extraction: Surfacing mentions of competitors during sales interactions
Impact on sales behavior: Sales teams have better insight into what messaging works, creating expectations for more data-driven content from marketing.
4. Intelligent Automation
AI streamlines the administrative aspects of selling:
- Automated CRM updates: Eliminating manual data entry after customer interactions
- Meeting scheduling optimization: Reducing the back-and-forth of appointment setting
- Follow-up automation: Ensuring consistent engagement without manual tracking
Impact on sales behavior: With the administrative burden reduced, sales teams can engage with more accounts and expect marketing support for a higher volume of opportunities.
Marketing’s Evolving Role in AI-Powered Sales Enablement
As sales teams adopt these AI capabilities, marketing’s approach to sales enablement must evolve in response:
1. From Static Content to Dynamic Content Systems
The shift: Traditional marketing-created sales assets (case studies, white papers, one-pagers) are too rigid for AI-powered personalization.
The new approach: Marketing must develop modular content systems with:
- Component-based design that allows for dynamic assembly
- Metadata tagging for intelligent content retrieval
- Variations addressing different buyer personas and stages
- Content designed for both human and AI consumption
Implementation example: Enterprise software company DocuSign redesigned its content strategy around a modular approach, creating component libraries rather than finished assets. This allowed their AI sales enablement platform to automatically assemble personalized presentations and proposals, increasing efficiency and effectiveness.
2. From Periodic Training to Continuous Enablement
The shift: Traditional quarterly sales training and periodic content updates are too slow for the AI-accelerated sales environment.
The new approach: Marketing needs to establish systems for:
- Real-time messaging updates based on market changes
- Continuous testing and optimization of sales narratives
- On-demand learning resources accessible at the point of need
- Feedback loops that capture field intelligence
Implementation example: Cybersecurity firm CrowdStrike implemented a “living playbook” approach where marketing continuously updates sales messaging based on AI analysis of successful sales conversations. This reduced the time to deploy updated messaging from weeks to days, giving sales a consistent competitive edge in a rapidly evolving market.
3. From Assumed Buyer Journeys to Data-Driven Engagement Maps
The shift: Marketing’s traditional buyer journey maps often fail to match the complex, non-linear paths that AI analytics reveal.
The new approach: Marketing teams must:
- Collaborate with sales on AI-detected engagement patterns
- Develop content mapped to actual (not theoretical) buying behaviors
- Create assets for specific micro-moments in the customer journey
- Design content for multi-channel, asynchronous buying processes
Implementation example: Industrial manufacturer Rockwell Automation used AI analysis of buyer engagement patterns to identify previously unknown research behaviors. Marketing responded by creating new content categories addressing discovery-stage technical evaluations that weren’t visible in traditional funnel models.
4. From Generic Insights to Actionable Intelligence
The shift: General market research and buyer personas are insufficient for AI-enabled sales teams accustomed to specific, actionable guidance.
The new approach: Marketing needs to provide:
- Account-specific competitive intelligence
- Industry micro-trend analysis
- Buying committee insight mapping
- Predictive content recommendations
Implementation example: Financial services technology provider FIS established a “Marketing Intelligence Desk” that combines AI market analysis with human expertise to deliver weekly account-specific guidance to sales teams. This approach increased competitive win rates by 17%, equipping sales with precisely targeted insights.
5. From Campaign Metrics to Revenue Intelligence
The shift: Traditional marketing metrics (impressions, clicks, downloads) don’t align with the revenue-focused analytics that AI provides to sales teams.
The new approach: Marketing measurement must evolve to include:
- Content effectiveness by sales stage
- Asset influence on deal velocity and size
- Messaging impact on competitive win rates
- Enablement ROI analysis
Implementation example: Healthcare technology company Philips implemented a unified revenue intelligence platform that tracks marketing content from creation through sales use to closed revenue. This connected data model increased marketing’s credibility with sales by demonstrating that marketing-influenced deals closed 27% faster than non-influenced opportunities.
The Technology Stack for AI-Powered Sales Enablement
Effective collaboration requires marketing teams to understand and integrate with the AI-powered tools that sales teams increasingly rely on:
Core Platform Categories
- Conversation Intelligence Platforms
- Analyze sales calls and meetings
- Extract insights from buyer-seller interactions
- Coach representatives based on successful patterns
- Examples: Gong, Chorus, ExecVision
- Revenue Intelligence Systems
- Connect data across the buyer journey
- Provide unified visibility into deal progression
- Offer predictive guidance on opportunity management
- Examples: Clari, InsightSquared, People.ai
- Sales Engagement Platforms
- Automate multi-channel outreach sequences
- Track prospect engagement across touchpoints
- Optimize cadence and messaging based on results
- Examples: Outreach, SalesLoft, Groove
- AI-Powered Sales Enablement Solutions
- Deliver the right content at the right time
- Provide guided selling based on deal context
- Offer real-time coaching during customer interactions
- Examples: Highspot, Seismic, Showpad
- Buyer Intent Platforms
- Identify accounts showing purchase interest
- Track digital body language across the web
- Alert sales to engagement opportunities
- Examples: 6sense, Demandbase, ZoomInfo
Integration Imperatives for Marketing
For marketing to effectively support sales enablement in this ecosystem:
- Establish bidirectional data flows between marketing automation and sales enablement platforms
- Ensure content management systems support the metadata requirements of AI-powered content delivery
- Align analytics frameworks to create a unified view of content performance across marketing and sales
- Develop API-first approaches that allow for flexible integration as the technology landscape evolves
Building Marketing Capabilities for AI-Powered Sales Enablement
Supporting sales in the AI era requires marketing teams to develop new competencies:
1. Content Strategy for Dynamic Assembly
Marketing teams need expertise in:
- Modular content architecture
- Component-based creation workflows
- Metadata taxonomy development
- Personalization rule design
Skill development approach: Partner with sales enablement platform vendors for specialized training and consider certification programs in adaptive content design.
2. Data Literacy and Analytics Expertise
Marketing teams need to understand:
- Natural language processing fundamentals
- Predictive analytics interpretation
- Machine learning model outcomes
- Revenue attribution modeling
Skill development approach: Implement paired learning between marketing and data science teams, with marketing team members shadowing analytics projects.
3. Sales Process Intelligence
Marketing teams should develop knowledge of:
- Sales methodology fundamentals
- Opportunity stage requirements
- Competitive positioning dynamics
- Objection handling frameworks
Skill development approach: Establish regular ride-along programs where marketing team members observe sales calls and meetings to understand frontline realities.
4. Agile Content Operations
Marketing teams need to implement:
- Rapid content development processes
- A/B testing frameworks for sales assets
- Continuous optimization workflows
- Cross-functional content sprints
Skill development approach: Adopt agile methodologies tailored explicitly for marketing operations, emphasizing sales enablement as a primary “customer.”
Implementation Roadmap: Marketing’s Path to AI-Enabled Sales Support
For marketing teams looking to enhance their sales enablement capabilities in the AI era, this phased approach provides a structured path forward:
Phase 1: Foundation Building (1-3 Months)
- Audit existing sales enablement content and processes
- Establish regular communication channels with sales leadership
- Inventory the AI tools already in use by the sales organization
- Identify immediate gaps in sales support materials
Phase 2: Pilot Implementation (3-6 Months)
- Select one high-impact sales scenario for AI-enhanced enablement
- Develop modular content for this specific use case
- Implement essential integration with sales AI platforms
- Establish a measurement framework for the pilot
Phase 3: Scaled Deployment (6-12 Months)
- Expand modular content approach across the full sales process
- Implement comprehensive integration with the sales tech stack
- Develop specialized roles focused on sales intelligence
- Create continuous feedback loops between systems
Phase 4: Advanced Optimization (12+ Months)
- Implement predictive content recommendations
- Develop autonomous content personalization capabilities
- Establish real-time optimization based on conversation intelligence
- Create unified revenue intelligence across marketing and sales
Common Pitfalls and How to Avoid Them
As marketing teams enhance their sales enablement capabilities, several common challenges emerge:
1. Tool Proliferation Without Integration
The problem: Implementing multiple AI platforms that don’t share data or insights.
The solution: Develop a unified data strategy before selecting new tools and prioritize integration capabilities in procurement decisions.
2. Content Volume Over Content Effectiveness
The problem: Creating numerous content variations without understanding which drive results.
The solution: Implement rigorous content testing and attribution to identify high-performing assets before scaling production.
3. Automating Broken Processes
The problem: Applying AI to dysfunctional sales and marketing workflows.
The solution: Map and optimize core processes before implementing AI enhancements.
4. Technical Capability Without Human Adoption
The problem: Deploying sophisticated AI tools that sales teams don’t understand or trust.
The solution: Involve sales representatives in solution design and implementation, focusing on practical value rather than technical sophistication.
Measuring Success: New Metrics for AI-Enhanced Sales Enablement
Traditional metrics fail to capture the impact of modern sales enablement. Consider these alternative measurements:
- Content Utilization Rate: Percentage of marketing assets used by sales
- Deal Influence Metrics: How marketing content impacts deal progression
- Time to Competency: How quickly new sales hires reach productivity
- Competitive Win Rate Impact: How enabled teams perform against competitors
- Revenue Efficiency: Revenue generated per sales hour worked
The Future of AI-Powered Sales and Marketing Alignment
Looking ahead, several emerging trends will further reshape sales enablement:
1. Autonomous Content Creation
AI will increasingly generate customized sales content in real time, requiring marketing to shift from content creation to content governance and strategy.
2. Digital Sales Rooms
Virtual spaces for buyer- seller collaboration will replace traditional content delivery, requiring marketing to design interactive experiences rather than static assets.
3. Ambient Intelligence
AI will provide real-time guidance during live customer interactions, requiring marketing to develop frameworks for in-the-moment support.
4. Unified Revenue Teams
Traditional boundaries between marketing, sales, and customer success will continue to blur, creating unified revenue teams aligned around customer lifetime value.
Conclusion
The rise of AI in sales enablement represents both a challenge and an opportunity for marketing teams. Those who understand these changes and evolve their approach will become indispensable strategic partners to sales. Those who don’t risk irrelevance in an increasingly AI-driven business environment.
By shifting from static content to dynamic systems, from periodic training to continuous enablement, from assumed buyer journeys to data-driven engagement maps, from generic insights to actionable intelligence, and from campaign metrics to revenue intelligence, marketing teams can thrive in this new ecosystem.
The future belongs to marketing teams that embrace their role as architects of intelligent sales enablement—designing the content, insights, and systems AI platforms use to empower the modern sales organization.
To learn how SkillSpot can help your business, visit www.theskillspot.com