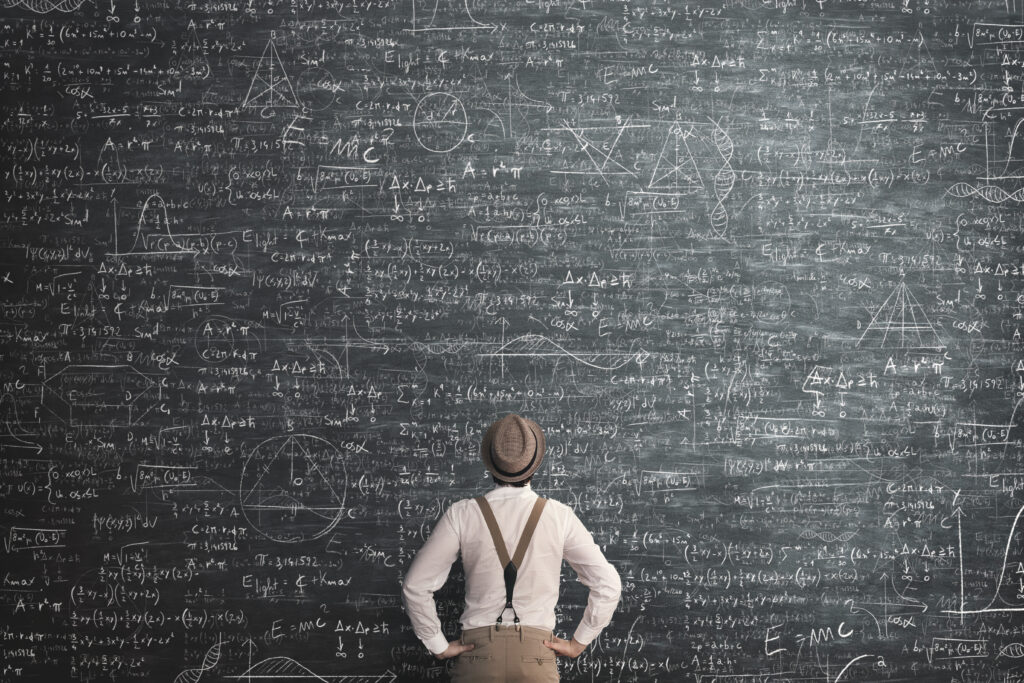
Most B2B organizations believe they understand their target customers. They’ve created detailed Ideal Customer Profiles (ICPs), developed buyer personas, and crafted messaging they think resonates with their market. Yet, despite these efforts, many still struggle with low conversion rates, extended sales cycles, and messaging that fails to drive action.
The uncomfortable truth is that your ICP and messaging are likely based more on internal assumptions and wishful thinking than on objective reality. This disconnect costs companies millions in wasted marketing spend, misdirected sales efforts, and missed revenue opportunities.
Fortunately, advances in artificial intelligence now provide unprecedented capabilities to validate, refine, and enhance your ICP and messaging through data-driven insights. This article will show you exactly how to leverage AI to transform these critical elements of your go-to-market strategy from educated guesses into precision instruments.
The Costly Delusion of “Knowing Your Customer”
Before exploring solutions, let’s examine why so many organizations operate with fundamentally flawed ICPs and messaging:
The Four Fatal Flaws in Traditional ICP Development
- Confirmation Bias: Teams naturally gravitate toward evidence that supports existing beliefs about their market.
- Success Sampling Error: Over-indexing on characteristics of a few successful customers while ignoring broader patterns.
- Historical Anchoring: Clinging to customer definitions that worked in the past but no longer reflect current market realities.
- Wishful Targeting: Defining ideal customers based on who teams want to sell to rather than who derives value from the solution.
The Messaging Mirage
Similarly, messaging often falls victim to:
- Inside-Out Thinking: Focusing on product features and company priorities rather than customer challenges and outcomes.
- Aspiration vs. Reality Gap: Developing messaging for an idealized buyer journey that bears little resemblance to how prospects purchase.
- Jargon Dependency: Relying on industry terminology that creates the illusion of precision while obscuring meaning.
- False Consensus Effect: Assuming customers value the same aspects of your solution that your internal teams do.
These fundamental issues can’t be solved simply by holding internal workshops or creating more detailed documentation. They require objective, data-driven validation and refinement—exactly what AI now makes possible.
The AI-Driven ICP Refinement System
Here’s a systematic approach to using AI for developing truly accurate Ideal Customer Profiles:
Phase 1: Data Aggregation and Pattern Recognition
Step 1: Comprehensive Customer Data Integration
Begin by consolidating data from multiple sources:
- CRM data (win rates, deal sizes, sales cycles)
- Customer success metrics (retention, expansion, NPS)
- Product usage analytics (adoption, feature utilization)
- Support and service interactions (ticket volumes, resolution times)
- Voice of customer data (surveys, interviews, feedback)
AI Application: Use data integration tools with AI capabilities to normalize and connect disparate data sets, creating a unified customer data foundation.
Implementation example:
Enterprise software company Acme Technologies consolidated five years of customer data from Salesforce, Gainsight, Pendo, Zendesk, and Qualtrics using Snowflake and Census. This created a unified customer dataset covering 4,200+ accounts with 142 potential ICP attributes.
Step 2: Multi-dimensional Success Pattern Analysis
Move beyond simplistic firmographic definitions to identify complex success patterns:
- Identify accounts with highest retention, growth, and satisfaction
- Apply machine learning to detect non-obvious correlation patterns
- Develop multi-factor success indicators beyond traditional firmographics
- Identify warning signals that predict customer misalignment
AI Application: Machine learning algorithms can identify patterns in customer success that humans would miss, particularly when simultaneously analyzing hundreds of potential variables.
Implementation example:
Using Amazon SageMaker, Acme's data science team built a customer success prediction model. Surprisingly, the strongest predictor of long-term success wasn't company size or industry, but the presence of a formal digital transformation initiative and cross-functional buying committees with 5+ members.
Step 3: Behavioral Segmentation
Traditional ICPs often define who should buy your product. AI-enhanced ICPs identify who does buy, implement, and succeed with your product:
- Analyze pre-sales engagement patterns that correlate with closed business
- Identify common implementation approaches among successful customers
- Map usage patterns that predict expansion and advocacy
- Discover segment-specific success factors and risk indicators
AI Application: AI can segment your customer base in ways that transcend traditional boundaries, revealing behavioral cohorts with similar success patterns, regardless of industry or size.
Implementation example:
Using clustering algorithms, Acme discovered three distinct customer segments that succeeded for different reasons:
1. "Efficiency Optimizers" - Focused on cost reduction, heavy integration with existing systems
2. "Experience Innovators" - Prioritized customer-facing improvements, rapid implementation
3. "Data Unifiers" - Valued reporting and analytics capabilities, methodical rollout
Each segment had different success metrics, implementation approaches, and growth patterns—requiring significantly different ICPs and messaging.
Phase 2: Market Intelligence Enhancement
AI doesn’t just analyze your internal data—it can enrich your understanding with external market insights:
Step 4: AI-Powered Market Research
Leverage AI to analyze vast amounts of industry content, social conversation, and competitive positioning:
- Monitor industry publications and analyst reports for emerging trends
- Track social media for unfiltered feedback about pain points
- Analyze job listings to identify changing priorities and investments
- Monitor competitor messaging and positioning evolution
AI Application: Natural language processing can analyze thousands of external documents to identify trends, pain points, and terminology that should inform your ICP and messaging.
Implementation example:
Using Crayon and AlphaSense with custom NLP models, Acme analyzed:
- 200+ industry analyst reports
- 15,000+ social media conversations about their product category
- 5,000+ relevant job postings from target accounts
- Messaging evolution from 12 competitors over 24 months
This analysis revealed three emerging pain points not addressed in their current messaging and identified a new buyer role gaining influence in purchase decisions.
Step 5: Voice of Customer Analysis at Scale
Use AI to derive deeper insights from customer interactions:
- Analyze sales call transcripts to identify practical discovery questions
- Extract common objections and concerns from prospect communications
- Identify language patterns between customer descriptions and official messaging
- Compare successful vs. unsuccessful sales conversations
AI Application: Conversation intelligence platforms with AI capabilities can extract patterns from thousands of customer interactions that would be impossible to identify manually.
Implementation example:
By analyzing 1,200+ sales call recordings with Gong's AI, Acme discovered:
- Prospects who asked about integration in the first 10 minutes closed at 3x higher rates
- Certain technical objections consistently predicted lost deals when raised by IT stakeholders
- Deals closed fastest when the phrase "operational visibility" appeared in early discovery
- Specific incumbent competitor mentions strongly correlated with longer sales cycles
These insights led to significant refinements in both their ICP (adding technical environment criteria) and messaging (emphasizing integration and operational visibility earlier).
Phase 3: ICP Validation and Refinement
With enhanced data and insights, it’s time to validate and refine your ICP:
Step 6: Predictive ICP Modeling
Move from descriptive to predictive ICP definitions:
- Develop AI models that predict customer success likelihood
- Create scoring mechanisms for prospect qualification
- Identify account expansion predictors
- Build churn risk indicators into the ICP
AI Application: Predictive modeling can transform your ICP from a static description into a dynamic evaluation tool.
Implementation example:
Acme developed a 100-point prospect scoring model using supervised machine learning, trained on their historical customer data. The model incorporated 27 factors including:
- Technical environment complexity (15 points)
- Digital transformation maturity (12 points)
- Cross-functional buying committee presence (10 points)
- Incumbent solution limitations (8 points)
- Executive sponsorship level (8 points)
- Implementation resource availability (7 points)
- Industry-specific regulatory pressures (5 points)
- Growth trajectory indicators (5 points)
Accounts scoring 70+ converted to customers at 4x the rate of those scoring below 40, and had 70% higher lifetime value.
Step 7: Continuous ICP Evolution
Implement systems for ongoing refinement:
- Establish automated feedback loops from sales, implementation, and success teams
- Set up regular AI-driven analysis cycles to identify changing patterns
- Create structured testing programs for ICP hypotheses
- Develop dashboards to monitor ICP alignment metrics
AI Application: AI systems can continuously monitor changing patterns in your customer data, alerting you to emerging trends before they become apparent.
Implementation example:
Acme implemented a quarterly ICP refresh cycle that:
1. Automatically aggregated new customer data
2. Re-ran predictive success models with recent data
3. Identified statistically significant changes in success factors
4. Flagged potential ICP adjustments for marketing and sales leadership review
5. Tracked the performance impact of previous ICP refinements
This system identified an emerging customer segment in manufacturing that wasn't previously part of their ICP but showed strong success indicators.
The AI-Enhanced Messaging Alignment System
With a refined ICP in place, AI can transform your messaging from assumption-based to evidence-driven:
Phase 1: Message Mining and Analysis
Step 1: Customer Language Harvesting
Use AI to analyze how customers describe their challenges, goals systematically, and your solution:
- Mine customer interviews, testimonials, and reviews for authentic language
- Analyze support tickets for problem description patterns
- Extract terminology from user forums and communities
- Compare internal language to customer-generated terms
AI Application: Natural language processing can identify patterns in how customers describe their situation versus how you describe your solution.
Implementation example:
Using MonkeyLearn and custom NLP tools, Acme analyzed:
- 75 customer testimonials and case studies
- 3,200+ support tickets
- 1,100+ community forum posts
- 800+ product reviews
The analysis revealed that while their messaging emphasized "workflow automation," customers consistently described the value as "eliminating information silos" and "reducing coordination overhead"—significantly different language for the same core benefit.
Step 2: Winning Message Identification
Analyze successful sales interactions to identify effective messaging patterns:
- Compare messaging used in won vs. lost deals
- Identify language patterns that accelerate buying processes
- Extract objection-handling approaches that resonate
- Detect message sequencing that drives commitment
AI Application: Conversation intelligence platforms can help identify which phrases and messages correlate with deal advancement.
Implementation example:
By analyzing successful sales emails and call transcripts, Acme discovered:
- Messaging that connected their solution to "operational resilience" resonated strongly with C-level stakeholders
- Stories about "time to insight" outperformed ROI-focused narratives by 3x
- Concrete examples of cross-departmental visibility drove more engagement than technical capability descriptions
- Middle managers responded best to messages about "reduced fire drills" and "predictable outcomes"
These insights led to a messaging matrix tailored to different stakeholders and buying stages.
Phase 2: Messaging Optimization and Testing
Step 3: AI-Driven Message Testing
Use AI to test and refine messaging systematically:
- Conduct large-scale A/B testing across digital channels
- Analyze email open and response rates by message variation
- Test messaging resonance across different segments
- Identify message-audience fit patterns
AI Application: AI can help design statistically valid tests and rapidly interpret results across multiple channels and segments.
Implementation example:
Acme implemented a systematic message testing program using:
- Email subject line and body testing (24 variations)
- Landing page message testing (12 variations)
- Ad copy testing (30+ variations)
- Sales outreach template testing (18 variations)
Results were analyzed by segment, stage, and stakeholder role. This identified that for their "Efficiency Optimizer" segment, messaging about "reduced manual reconciliation" generated 3.4x higher engagement than their standard value proposition.
Step 4: Competitive Differentiation Analysis
Use AI to ensure your messaging stands out from competitors:
- Track competitor messaging evolution across channels
- Identify overcrowded message territories
- Discover underutilized differentiation opportunities
- Test relative message impact against competitive alternatives
AI Application: AI can continuously monitor competitor messaging across channels to identify saturation and opportunity areas.
Implementation example:
Using Crayon's competitive intelligence platform with custom analysis, Acme tracked messaging from 8 key competitors across websites, emails, social, and advertising. They discovered:
- 5 competitors used nearly identical messaging around "360-degree visibility"
- Only 2 competitors emphasized implementation speed, despite this being highly valued
- No competitors specifically addressed cross-departmental collaboration challenges
- All competitors focused on features rather than business outcomes in technical materials
This analysis helped Acme develop truly differentiated messaging that avoided crowded territory while emphasizing validated customer priorities that competitors ignored.
Phase 3: Persona-Specific Messaging Alignment
Step 5: Stakeholder-Specific Message Mapping
Develop precisely calibrated messaging for each buying committee member:
- Create role-specific language and frameworks
- Align messages to role-specific success metrics
- Develop stakeholder-specific objection responses
- Map messages to buying journey stages by role
AI Application: AI can analyze which messages resonate with specific roles and suggest optimal messaging for stakeholders.
Implementation example:
Acme developed a stakeholder message matrix that mapped:
- 5 key buyer roles (CIO, COO, VP of Operations, IT Director, Business Analyst)
- 4 buying stages (Problem Awareness, Solution Exploration, Vendor Selection, Decision Validation)
- 3 primary use cases (Process Optimization, Cross-Functional Coordination, Operational Intelligence)
For each intersection, they identified:
- Primary pain points and desired outcomes
- Key objections and concerns
- Proof points and validation needs
- Optimal content formats and channels
This matrix was embedded in their sales enablement platform, automatically suggesting the right messaging based on prospect role and stage.
Step 6: Messaging Effectiveness Measurement
Implement systems to track and improve message performance:
- Set up message attribution tracking across the buying journey
- Measure message resonance by channel and format
- Track message effectiveness at advancing buying stages
- Analyze message memorability and internal propagation
AI Application: Attribution modeling with AI can help understand which messages drive buying decisions across complex journeys.
Implementation example:
Using multi-touch attribution modeling, Acme tracked which messages influenced pipeline progression:
- Messages about "operational resilience" generated 2x the MQLs but converted to customers at half the rate of messages about "cross-functional visibility"
- Technical stakeholders exposed to integration messaging early in the journey shortened sales cycles by 40%
- Accounts that engaged with ROI content converted at similar rates but had 2.4x higher initial contract values
- Messages delivered through industry webinars drove higher-quality opportunities than identical messages in direct channels
These insights helped refine not just what messages to use, but when and how to deliver them for maximum impact.
Implementation: Your 60-Day AI-Enhanced ICP and Messaging Roadmap
Here’s a practical implementation plan for organizations ready to enhance their ICP and messaging with AI:
Phase 1: Foundation and Discovery (Days 1-15)
Week 1: Data Preparation
- Inventory available customer data sources
- Identify initial AI tools for analysis (start with what you have access to)
- Create a unified customer dataset for analysis
- Establish success metrics for the initiative
Week 2: Initial Analysis
- Run preliminary AI analysis on customer success patterns
- Gather and analyze external data (competitor messaging, industry content)
- Conduct initial voice of customer analysis
- Document gaps between current ICP/messaging and initial findings
Phase 2: Insight Development (Days 16-30)
Week 3: Deep Analysis
- Develop comprehensive success pattern analysis
- Create behavioral segments based on usage and outcomes
- Analyze sales conversation data for message effectiveness
- Identify preliminary ICP refinements
Week 4: Validation and Testing
- Design validation tests for ICP hypotheses
- Develop messaging variations based on customer language
- Create segment-specific value propositions
- Begin message testing across digital channels
Phase 3: Implementation and Optimization (Days 31-60)
Week 5-6: Operationalization
- Refine ICP definition and scoring model
- Develop stakeholder-specific messaging frameworks
- Create updated messaging guidelines and playbooks
- Configure attribution systems for ongoing measurement
Week 7-8: Activation and Measurement
- Train marketing and sales teams on refined ICP and messaging
- Implement new messaging across channels
- Establish continuing measurement and optimization process
- Create a dashboard for tracking impact metrics
Case Study: AI-Driven ICP and Messaging Transformation
Company: Enterprise SaaS provider in operations management Challenge: Stagnant growth, low conversion rates, extended sales cycles
Approach:
- Implemented comprehensive AI analysis of customer success patterns
- Analyzed 3,000+ sales conversations for message effectiveness
- Developed predictive ICP scoring model with 22 factors
- Created stakeholder-specific messaging for four buying roles
- Established continuous testing and optimization process
Results:
- 41% increase in marketing qualified leads to opportunity conversion
- 28% reduction in average sales cycle
- 63% improvement in cold outreach response rates
- 37% higher win rates against key competitors
- 24% increase in average contract value
Key Learning: “We discovered our actual ideal customers fundamentally differed from who we thought they were. More importantly, the language they used to describe their challenges had almost no overlap with our messaging. AI helped us close these gaps with precision that would have been impossible through traditional methods.”
Common Pitfalls and Solutions
As you implement AI-enhanced ICP and messaging, watch for these common challenges:
Pitfall 1: Data Quality Issues
Problem: Incomplete or inconsistent customer data undermines AI analysis.
Solution: Begin with a data quality assessment and improvement project. Even with imperfect data, start with the highest-quality segments while implementing better data capture processes.
Pitfall 2: Over-complexity
Problem: Creating overly complex ICP models that are difficult to operationalize.
Solution: Focus on the 5-7 most predictive factors for initial implementation, then add sophistication over time as adoption increases.
Pitfall 3: Resistance to Change
Problem: Sales and marketing teams resist adopting new ICP definitions and messaging.
Solution: Involve front-line teams in the discovery process, sharing compelling evidence directly addressing their pain points. Demonstrate early wins through controlled testing.
Pitfall 4: Analysis Paralysis
Problem: Continuous analysis without decisive implementation.
Solution: Establish clear decision thresholds and implementation timelines. Perfect insight is impossible, but significant improvement is achievable quickly.
Pitfall 5: Tool Fragmentation
Problem: Using too many disconnected AI tools without integration.
Solution: Start with a core set of integrated tools, focusing on high-impact use cases before expanding. Prioritize tools that work with your existing stack.
Conclusion: The Competitive Advantage of AI-Enhanced Understanding
The gap between companies’ ideal customers and their actual customers remains one of the most costly disconnects in B2B marketing and sales. Similarly, the difference between how companies talk about their solutions and how customers actually perceive value represents millions in wasted spending and lost opportunity.
AI now provides unprecedented capability to close these gaps—not through more assumptions but through objective, data-driven analysis of what works. Organizations that systematically apply these capabilities gain a fundamental competitive advantage: they focus resources on actual ideal customers and communicate in ways that genuinely resonate.
The companies dominating their markets in the coming years won’t necessarily be those with the best products or the largest sales forces. They’ll be those with the most precise understanding of their customers—an understanding increasingly impossible to achieve without AI-enhanced approaches to ICP definition and messaging development.
The question isn’t whether your current ICP and messaging have room for improvement—they certainly do. The question is whether you’ll leverage AI to make those improvements before your competitors.
To learn how SkillSpot can help your business, visit www.theskillspot.com