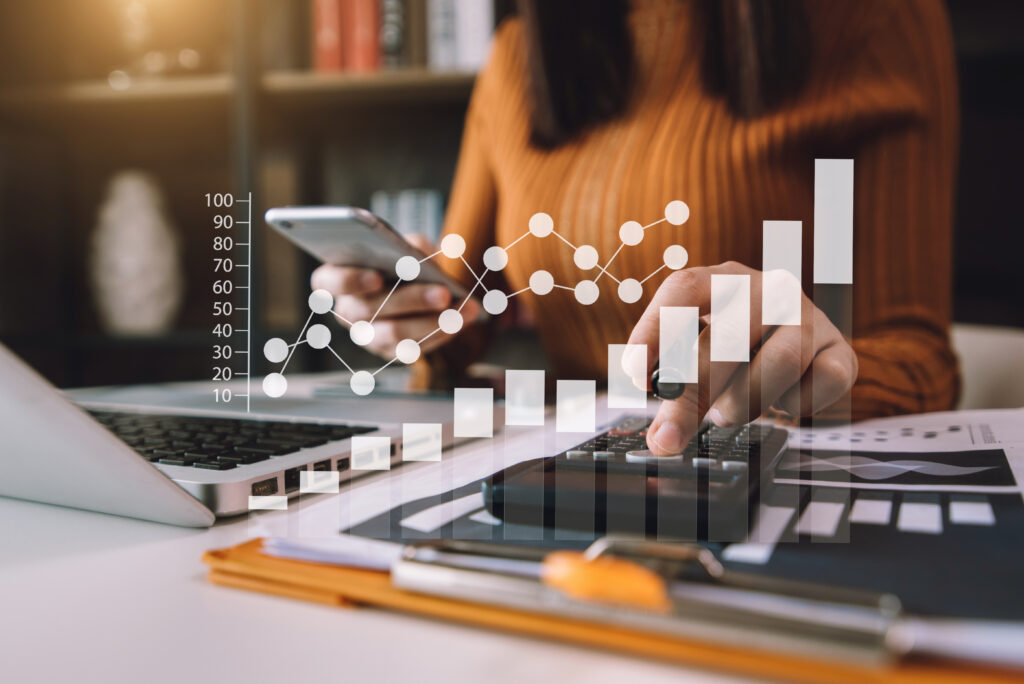
The traditional B2B sales funnel is dead.
Today’s buyers don’t follow a linear path from awareness to decision. They research independently, engage across multiple channels, loop back through various stages, and involve numerous stakeholders before making purchasing decisions. This complex reality requires a fundamentally different approach to sales funnel design and management.
Artificial intelligence has emerged as the critical technology enabling sales organizations to adapt to this new landscape. By leveraging AI throughout the buyer journey, forward-thinking companies are creating intelligent, responsive sales funnels that meet buyers where they are, anticipate their needs, and guide them toward decisions in ways that feel helpful rather than pushy.
This guide will show you exactly how to map the modern buyer journey and implement AI at each stage to create a sales funnel that converts more prospects with less wasted effort.
The Modern B2B Buyer Journey: What’s Changed
Before building your AI-enhanced funnel, you need to understand how B2B buying has evolved:
Key Shifts in B2B Buying Behavior
- Self-Directed Research Dominates
- Buyers complete 70-80% of their journey before contacting sales
- 62% rely on web searches; 56% on vendor websites; 42% on peer recommendations
- 72% of buyers expect personalized engagement based on their specific needs
- Multiple Stakeholders Complicate Decisions
- Average of 6-10 decision-makers involved in B2B purchases
- 77% of buyers describe their process as very complex or challenging
- Each stakeholder researches independently, creating asynchronous journeys
- Non-Linear Progression Is Standard
- 90% of buyers report looping back to previous stages at least once
- Average buyer consults 5-8 information sources before purchase
- Decision timeline has extended 22% in the past five years
- Digital and Human Touchpoints Intertwine
- 59% of buyers prefer remote interactions; 41% want in-person engagement
- 83% of B2B buyers prefer ordering or paying through digital commerce
- 76% find it helpful to speak to someone when buying a completely new solution
These shifts require a funnel that is responsive, personalized, and intelligent—precisely what AI enables when properly implemented.
Mapping Your Modern Sales Funnel
Before applying AI, you need a clear map of your actual buyer journey, not an idealized version of it:
Step 1: Identify Your Buyer Journey Stages
Modern B2B funnels typically include these stages, though terminology may vary:
- Problem Recognition
- Buyer recognizes a challenge or opportunity
- Begins preliminary, often anonymous research
- Determines if the problem is worth solving
- Solution Exploration
- Investigates potential approaches to solving the problem
- Evaluates build vs. buy options
- Develops initial requirements and criteria
- Vendor Consideration
- Identifies potential solution providers
- Conducts comparison research
- Engages with sales or requests information
- Stakeholder Alignment
- Builds internal consensus
- Addresses department-specific concerns
- Develops formal business case
- Validation and Selection
- Conducts detailed evaluation (demos, trials, etc.)
- Negotiates terms and conditions
- Makes final selection
- Implementation Planning
- Prepares for deployment
- Addresses technical and organizational requirements
- Establishes success metrics
Step 2: Map Stakeholder-Specific Journeys
Different buying committee members follow unique paths:
- Economic Buyers: Focus on ROI, strategic alignment, and business outcomes
- Technical Evaluators: Concentrate on capabilities, integrations, and implementation
- End Users: Prioritize usability, workflow impact, and practical functionality
- Procurement: Emphasizes pricing, terms, compliance, and vendor viability
Step 3: Identify Engagement Channels by Stage
Document where and how buyers engage throughout their journey:
- Digital channels: Website, social media, search engines, review sites, communities
- Content touchpoints: Blogs, whitepapers, case studies, webinars, comparison guides
- Interactive elements: Product tours, calculators, assessments, configurators
- Human interactions: Sales calls, demos, consultations, customer references
Step 4: Map Decision Criteria and Questions
For each stage and stakeholder, identify:
- Key questions buyers are trying to answer
- Evaluation criteria they’re likely applying
- Information sources they typically consult
- Objections and concerns that commonly arise
This comprehensive mapping creates the foundation for applying AI effectively throughout your funnel.
The AI-Enhanced Sales Funnel: Stage-by-Stage Implementation
Now let’s explore exactly how to implement AI at each stage of the modern B2B sales funnel:
Stage 1: Problem Recognition
At this stage, prospects are recognizing challenges but often haven’t formulated clear solution criteria. AI can help identify and engage these early-stage buyers:
AI Applications for Problem Recognition:
- Intent Signal Detection Technology: Buyer intent platforms with AI analysis Implementation:
- Deploy tools like 6sense, Bombora, or Demandbase to analyze behavioral signals
- Create scoring models for early-stage research behaviors
- Trigger appropriate engagement based on intent strength
- Predictive Account Identification Technology: Predictive analytics and machine learning Implementation:
- Build models that identify accounts showing early problem indicators
- Create “look-alike” targeting based on your successful customer patterns
- Prioritize outreach to accounts with highest likelihood of need
- AI-Powered Content Personalization Technology: Dynamic content platforms with machine learning Implementation:
- Create adaptive website experiences based on visitor signals
- Develop industry and role-specific content paths
- Personalize early-stage resources to specific challenges
Problem Recognition Measurement Framework:
Track these metrics to evaluate AI effectiveness at this stage:
- Intent signal accuracy (% of identified accounts that enter funnel)
- Content relevance scores (engagement with personalized content)
- Early-stage conversion rates (anonymous to known visitors)
- Problem awareness content performance by segment
Stage 2: Solution Exploration
As prospects begin investigating potential approaches, AI can guide their learning journey and capture engagement data:
AI Applications for Solution Exploration:
- Intelligent Content Recommendations Technology: Content recommendation engines Implementation:
- Implement AI-driven resource suggestions based on consumption patterns
- Develop “next best content” algorithms for resource pages
- Create content journeys that adapt to engagement signals
- Conversational AI for Research Guidance Technology: Advanced chatbots and virtual assistants Implementation:
- Deploy solution advisor chatbots to help prospects explore options
- Create interactive assessment tools that provide personalized guidance
- Develop problem-focused conversational flows
- Behavioral Analysis for Journey Mapping Technology: Machine learning for user journey analysis Implementation:
- Track and analyze content consumption sequences
- Identify common pathways that lead to conversion
- Optimize content journeys based on successful patterns
Solution Exploration Measurement Framework:
Key metrics for this stage include:
- Content journey completion rates
- Self-education depth (number of resources consumed)
- Engagement to inquiry conversion rate
- Solution consideration set inclusion
Stage 3: Vendor Consideration
As prospects begin evaluating specific solutions, AI helps position your offering effectively against alternatives:
AI Applications for Vendor Consideration:
- Competitive Intelligence and Positioning Technology: AI-powered competitive analysis platforms Implementation:
- Deploy tools to track competitor positioning and messaging
- Create adaptive positioning based on which competitors are being considered
- Develop “battle card” content that addresses specific competitive scenarios
- Predictive Lead Scoring and Qualification Technology: AI-based lead scoring models Implementation:
- Implement multi-factor scoring that goes beyond demographic criteria
- Create dynamic lead routing based on fit and engagement
- Develop separate scoring models for different product lines or segments
- Personalized Outreach Automation Technology: AI writing assistance and engagement platforms Implementation:
- Create personalized outreach templates based on prospect behaviors
- Implement timing optimization for sales engagement
- Develop multi-channel orchestration based on response patterns
Vendor Consideration Measurement Framework:
Track these metrics to evaluate effectiveness:
- Competitive win/loss improvements
- Lead scoring accuracy (predicted vs. actual conversion)
- Personalized outreach response rates
- Consideration set inclusion and progression
Stage 4: Stakeholder Alignment
As buying committees evaluate solutions, AI helps navigate complex multi-stakeholder dynamics:
AI Applications for Stakeholder Alignment:
- Buying Committee Intelligence Technology: Relationship intelligence and organizational mapping Implementation:
- Deploy tools to identify committee members and reporting structures
- Create stakeholder-specific content and engagement strategies
- Develop champions within accounts based on engagement patterns
- Consensus-Building Content and Tools Technology: Interactive content platforms with collaboration features Implementation:
- Create sharable assessment tools and business case generators
- Develop stakeholder-specific value propositions and content
- Implement analytics to track internal content sharing patterns
- Internal Selling Enablement Technology: Content activation and sharing analytics Implementation:
- Provide champions with AI-curated content for internal sharing
- Track internal circulation of shared materials
- Create micro-content designed for internal forwarding
Stakeholder Alignment Measurement Framework:
Evaluate effectiveness with these metrics:
- Multi-stakeholder engagement rates
- Internal content sharing analytics
- Champion activation success
- Decision committee coverage (% of committee engaged)
Stage 5: Validation and Selection
As prospects move toward decisions, AI helps address final concerns and facilitate the selection process:
AI Applications for Validation and Selection:
- Objection Anticipation and Response Technology: Predictive analytics and content recommendation Implementation:
- Analyze historical deal patterns to predict likely objections
- Create personalized content addressing specific concerns
- Implement objection resolution tracking
- Proposal and Deal Optimization Technology: AI-powered CPQ (Configure, Price, Quote) systems Implementation:
- Create optimal package recommendations based on stakeholder priorities
- Implement dynamic pricing models based on value-drivers
- Develop competitive positioning specific to final-stage alternatives
- Success Prediction and Risk Mitigation Technology: Implementation risk analysis and success modeling Implementation:
- Develop models to identify potential implementation challenges
- Create risk mitigation plans for high-risk factors
- Implement success planning tools for prospects
Validation and Selection Measurement Framework:
Key metrics include:
- Late-stage conversion improvements
- Objection resolution effectiveness
- Proposal acceptance rates
- Deal cycle time reduction
Stage 6: Implementation Planning
After selection but before implementation, AI helps ensure successful deployment:
AI Applications for Implementation Planning:
- Customer Onboarding Optimization Technology: AI-driven implementation planning tools Implementation:
- Create personalized onboarding plans based on customer characteristics
- Develop resource recommendations based on similar implementations
- Implement risk prediction for onboarding challenges
- Success Prediction and Enablement Technology: Predictive customer success modeling Implementation:
- Create adoption and value realization predictions
- Implement early warning systems for potential challenges
- Develop success playbooks based on data from similar customers
- Cross-Sell/Upsell Opportunity Identification Technology: Expansion opportunity modeling Implementation:
- Identify likely expansion paths based on initial implementation
- Develop staged growth plans for customer value journey
- Implement triggers for expansion opportunity signals
Implementation Planning Measurement Framework:
Track these metrics to evaluate effectiveness:
- Implementation readiness scores
- Time-to-value improvements
- Early adoption rates
- Initial expansion conversion
Building Your AI-Enhanced Funnel: Implementation Roadmap
To implement an AI-enhanced sales funnel effectively, follow this phased approach:
Phase 1: Foundation Building (1-2 Months)
- Journey Mapping and Data Assessment
- Document your current buyer journey with stakeholder input
- Audit available data sources and identify gaps
- Establish baseline metrics for current funnel performance
- Define success criteria for AI implementation
- Technology Stack Evaluation
- Assess current martech and salestech capabilities
- Identify priority AI applications for your specific needs
- Evaluate build vs. buy options for key functionality
- Develop integration requirements
- Pilot Planning
- Select 1-2 high-impact stages for initial AI implementation
- Define specific use cases and expected outcomes
- Create measurement framework for pilot evaluation
- Develop change management approach
Phase 2: Initial Implementation (2-3 Months)
- Data Integration and Preparation
- Connect relevant data sources for selected AI applications
- Clean and normalize historical data
- Create data governance framework
- Implement tracking for new data points
- Pilot Deployment
- Implement selected AI applications in controlled environment
- Train sales and marketing teams on new capabilities
- Develop process documentation and playbooks
- Establish feedback mechanisms
- Initial Optimization
- Analyze early results against baseline metrics
- Refine AI models based on performance data
- Address operational challenges
- Document lessons learned and adjust approach
Phase 3: Scaling and Enhancement (3-6 Months)
- Expanded Implementation
- Roll out proven AI applications across additional funnel stages
- Implement more sophisticated use cases
- Develop integrated workflows across applications
- Create comprehensive training program
- Advanced Analytics Development
- Implement cross-funnel analytics
- Create unified customer journey views
- Develop predictive pipeline models
- Establish revenue intelligence dashboards
- Continuous Improvement Framework
- Implement systematic testing and optimization
- Develop AI model refresh cadence
- Create innovation process for new use cases
- Establish centers of excellence
Overcoming Common Challenges
As you implement your AI-enhanced funnel, you’ll likely encounter these challenges:
Challenge 1: Data Quality and Integration
Problem: Disconnected data sources and inconsistent information undermine AI effectiveness.
Solution: Start with a data audit and quality improvement initiative. Focus initially on enhancing data in a few key areas rather than trying to fix everything at once. Implement progressive data enrichment processes that improve quality over time.
Challenge 2: Cross-Functional Alignment
Problem: Siloed operations between marketing, sales, and customer success create funnel gaps.
Solution: Create a revenue operations function that spans traditional departments. Develop shared metrics and goals that encourage collaboration. Implement technology that provides visibility across the entire customer journey.
Challenge 3: User Adoption
Problem: Sales teams resist adopting new AI-powered tools and processes.
Solution: Focus on solving real pain points rather than implementing technology for its own sake. Create clear “what’s in it for me” value propositions for users. Develop champions who can demonstrate success and coach others.
Challenge 4: Ethical Use of AI
Problem: Concerns about privacy, transparency, and ethical use of AI-generated insights.
Solution: Develop clear guidelines for ethical AI use. Maintain transparency with prospects about data usage. Implement governance processes that ensure AI applications align with company values and regulatory requirements.
Case Study: AI-Enhanced Funnel Transformation
Company: Enterprise software provider specializing in supply chain management Challenge: Lengthy sales cycles, low conversion rates, inefficient resource allocation
Approach:
- Implemented comprehensive buyer journey mapping across stakeholders
- Deployed AI-driven intent monitoring and predictive lead scoring
- Created personalized content journeys based on engagement patterns
- Developed AI-powered competitive positioning for late-stage deals
- Implemented success prediction and risk mitigation in final stages
Results:
- 43% reduction in average sales cycle
- 67% increase in marketing-sourced pipeline
- 28% improvement in lead-to-opportunity conversion
- 35% higher win rates against primary competitors
- 22% increase in average deal size
Key Learning: “The most valuable insight was discovering how non-linear our actual buyer journey was. Our traditional funnel model was causing us to miss key engagement opportunities. AI helped us meet prospects where they actually were in their journey, rather than where we thought they should be.”
The Future of AI in B2B Sales Funnels
As you implement your AI-enhanced funnel, keep these emerging trends in mind:
1. Autonomous Engagement
AI will increasingly manage early-stage prospect engagement independently, with human sales involvement coming later in the process and focusing on high-value activities.
2. Hyper-Personalization at Scale
Advances in AI will enable truly individualized experiences across all touchpoints, moving beyond basic personalization to experiences that adapt in real-time to prospect behavior.
3. Unified Revenue Intelligence
AI will bridge traditional gaps between marketing, sales, and customer success, creating seamless visibility and coordinated action across the entire customer lifecycle.
4. Augmented Sales Decision-Making
AI will increasingly provide real-time guidance to sales professionals, helping them navigate complex deals with recommendations based on patterns from thousands of similar situations.
Conclusion: The Competitive Advantage of an AI-Enhanced Funnel
The B2B sales landscape has fundamentally changed. Buyers are more informed, more demanding, and follow increasingly complex, non-linear journeys. Traditional sales funnels simply can’t adapt fast enough to these realities.
AI provides the intelligence, adaptability, and scale needed to transform your sales funnel from a rigid process into a responsive system that meets buyers where they are, anticipates their needs, and guides them toward decisions through value rather than pressure.
The companies that will dominate B2B sales in the coming years won’t necessarily be those with the largest sales forces or marketing budgets. They’ll be those who most effectively harness AI to understand, engage, and guide their prospects through increasingly complex buying journeys.
By methodically implementing AI across your sales funnel as outlined in this guide, you’ll not only improve conversion rates and efficiency but create a sustainable competitive advantage that becomes stronger over time as your AI applications learn and improve.
The future of B2B sales belongs to organizations that successfully blend human expertise with artificial intelligence—creating sales experiences that are simultaneously more efficient for sellers and more valuable for buyers.
To learn how SkillSpot can help your business, visit www.theskillspot.com